Technology can make it look as if anyone has said or done anything. Is it the next wave of (mis)information warfare?
In May 2018, a video appeared on the internet of Donald Trump offering advice to the people of Belgium on the issue of climate change. “As you know, I had the balls to withdraw from the Paris climate agreement,” he said, looking directly into the camera, “and so should you.”
The video was created by a Belgian political party, Socialistische Partij Anders, or sp.a, and posted on sp.a’s Twitter and Facebook. It provoked hundreds of comments, many expressing outrage that the American president would dare weigh in on Belgium’s climate policy.
But this anger was misdirected. The speech, it was later revealed, was nothing more than a hi-tech forgery.
Sp.a claimed that they had commissioned a production studio to use machine learning to produce what is known as a “deep fake” – a computer-generated replication of a person, in this case Trump, saying or doing things they have never said or done.
Sp.a’s intention was to use the fake video to grab people’s attention, then redirect them to an online petition calling on the Belgian government to take more urgent climate action. The video’s creators later said they assumed that the poor quality of the fake would be enough to alert their followers to its inauthenticity. “It is clear from the lip movements that this is not a genuine speech by Trump,” a spokesperson for sp.a told Politico.
As it became clear that their practical joke had gone awry, sp.a’s social media team went into damage control. “Hi Theo, this is a playful video. Trump didn’t really make these statements.” “Hey, Dirk, this video is supposed to be a joke. Trump didn’t really say this.”
The party’s communications team had clearly underestimated the power of their forgery, or perhaps overestimated the judiciousness of their audience. Either way, this small, left-leaning political party had, perhaps unwittingly, provided a deeply troubling example of the use of manipulated video online in an explicitly political context.
It was a small-scale demonstration of how this technology might be used to threaten our already vulnerable information ecosystem – and perhaps undermine the possibility of a reliable, shared reality.
Expert opinions
Danielle Citron, a professor of law at the University of Maryland, along with her colleague Bobby Chesney, began working on a report outlining the extent of the potential danger. As well as considering the threat to privacy and national security, both scholars became increasingly concerned that the proliferation of deep fakes could catastrophically erode trust between different factions of society in an already polarized political climate.
In particular, they could foresee deep fakes being exploited by purveyors of “fake news”. Anyone with access to this technology – from state-sanctioned propagandists to trolls – would be able to skew information, manipulate beliefs, and in so doing, push ideologically opposed online communities deeper into their own subjective realities.
“The marketplace of ideas already suffers from truth decay as our networked information environment interacts in toxic ways with our cognitive biases,” the report reads. “Deep fakes will exacerbate this problem significantly.”
Citron and Chesney are not alone in these fears. In April 2018, the film director Jordan Peele and BuzzFeed released a deep fake of Barack Obama calling Trump a “total and complete dipshit” to raise awareness about how AI-generated synthetic media might be used to distort and manipulate reality.
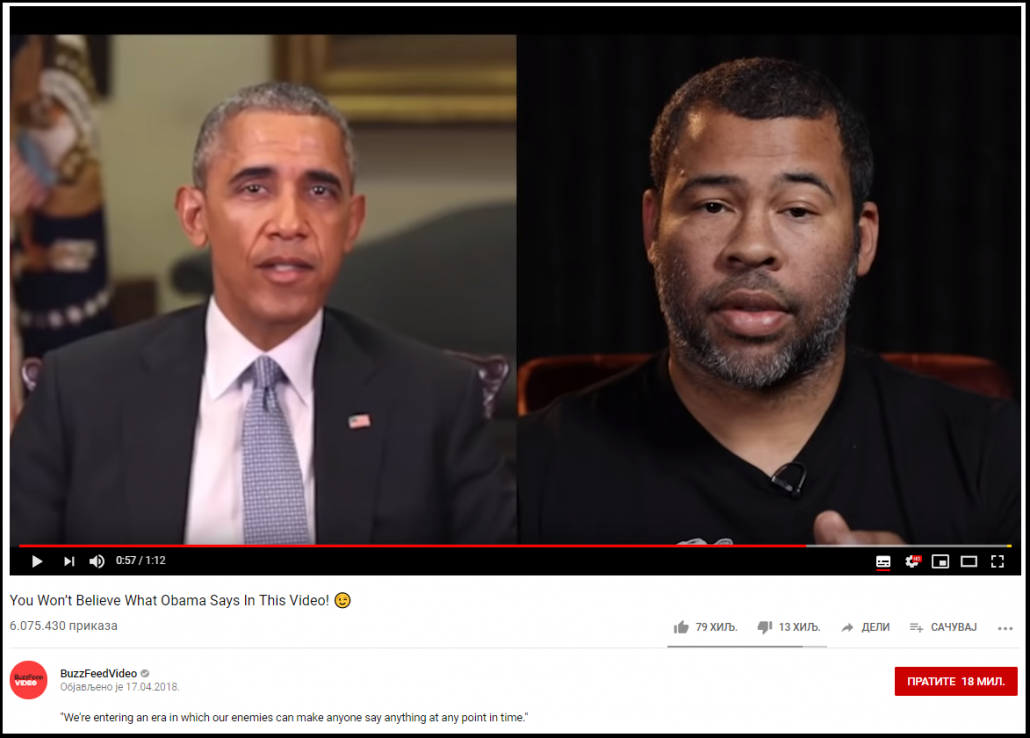
In September 2018, three members of Congress sent a letter to the director of national intelligence, raising the alarm about how deep fakes could be harnessed by “disinformation campaigns in our elections”.
While these disturbing hypotheticals might be easy to conjure, Tim Hwang, director of the Harvard-MIT Ethics and Governance of Artificial Intelligence Initiative, is not willing to bet on deep fakes having a high impact on elections in the near future. Hwang has been studying the spread of misinformation on online networks for a number of years, and, with the exception of the small-stakes Belgian incident, he is yet to see any examples of truly corrosive incidents of deep fakes “in the wild”.
Hwang believes that this is partly because using machine learning to generate convincing fake videos still requires a degree of expertise and lots of data. “If you are a propagandist, you want to spread your work as far as possible with the least amount of effort,” he said. “Right now, a crude Photoshop job could be just as effective as something created with machine learning.”
At the same time, Hwang acknowledges that as deep fakes become more realistic and easier to produce in the coming years, they could usher in an era of forgery qualitatively different from what we have seen before. In the past, for example, if you wanted to make a video of the president saying something he didn’t say, you needed a team of experts. Whereas today machine learning will not only automate this process, it will also probably make better forgeries.
Couple this with the fact that access to this technology will spread over the internet, and suddenly you have, as Hwang put it, “a perfect storm of misinformation”.
Technology on the rise
Nonetheless, research into machine learning-powered synthetic media forges ahead.
To make a convincing deep fake you usually need a neural model that is trained with a lot of reference material. Generally, the larger your dataset of photos, video, or sound, the more eerily accurate the result will be. But this May, researchers at Samsung’s AI Center in Moscow have devised a method to train a model to animate with an extremely limited dataset: just a single photo, and the results are surprisingly good.
The researchers were able to create the “photorealistic talking head models” using convolutional neural networks: they trained the algorithm on a large dataset of talking head videos with a wide variety of appearances. In this case, they used the publicly available VoxCeleb databases containing more than 7,000 images of celebrities from YouTube videos.
This trains the program to identify what they call “landmark” features of the faces: eyes, mouth shapes, the length and shape of a nose bridge.
This, in a way, is a leap beyond what even deep fakes and other algorithms using generative adversarial networks can accomplish. Instead of teaching the algorithm to paste one face onto another using a catalogue of expressions from one person, they use the facial features that are common across most humans to then puppeteer a new face.
As the team proves, its model even works on the Mona Lisa, and other single-photo still portraits. In the video, famous portraits of Albert Einstein, Fyodor Dostoyevsky, and Marilyn Monroe come to life as if they’re Live Photos in your iPhone’s camera roll. But like with most deep fakes, it’s pretty easy to see the seams at this stage. Most of the faces are surrounded by visual artifacts.
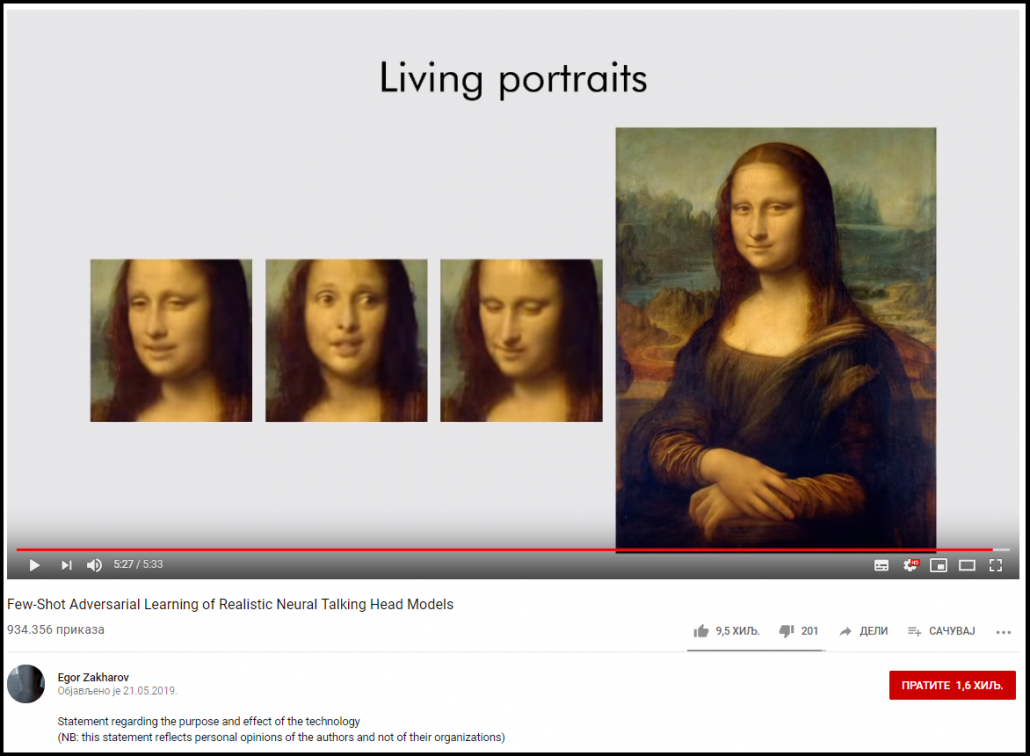
New detection methods
As the threat of deep fakes intensifies, so do efforts to produce new detection methods. In June 2018, researchers from the University at Albany (SUNY) published a paper outlining how fake videos could be identified by a lack of blinking in synthetic subjects. Facebook has also committed to developing machine learning models to detect deep fakes.
But Hany Farid, professor of computer science at the University of California, Berkeley, is wary. Relying on forensic detection alone to combat deep fakes is becoming less viable, he believes, due to the rate at which machine learning techniques can circumvent them. “It used to be that we’d have a couple of years between coming up with a detection technique and the forgers working around it. Now it only takes two to three months.”
This, he explains, is due to the flexibility of machine learning. “All the programmer has to do is update the algorithm to look for, say, changes of color in the face that correspond with the heartbeat, and then suddenly, the fakes incorporate this once imperceptible sign.”
Although Farid is locked in this technical cat-and-mouse game with deep fake creators, he is aware that the solution does not lie in new technology alone. “The problem isn’t just that deep fake technology is getting better,” he said. “It is that the social processes by which we collectively come to know things and hold them to be true or untrue are under threat.”
Reality apathy
Indeed, as the fake video of Trump that spread through social networks in Belgium demonstrated – a video for which it was later revealed that it was not forged by machine learning technology, as sp.a claimed at first, but by using an editing software called After Effects – deep fakes don’t need to be undetectable or even convincing to be believed and do damage. It is possible that the greatest threat posed by deep fakes lies not in the fake content itself, but in the mere possibility of their existence.
This is a phenomenon that scholar Aviv Ovadya has called “reality apathy”, whereby constant contact with misinformation compels people to stop trusting what they see and hear. In other words, the greatest threat isn’t that people will be deceived, but that they will come to regard everything as deception.
Recent polls indicate that trust in major institutions and the media is dropping. The proliferation of deep fakes, Ovadya says, is likely to exacerbate this trend.
According to Danielle Citron, we are already beginning to see the social ramifications of this epistemic decay. “Ultimately, deep fakes are simply amplifying what I call the liar’s dividend,” she said. “When nothing is true then the dishonest person will thrive by saying what’s true is fake.”